Data-driven Machine Learning Approach to Model and Control Aerospace systems for improved Aircraft performance and efficiency
PI: Dr. Ayman Abdallah
Co-I: Dr. Mohammed Ismail
This research explores the application of advanced machine learning techniques to derive symbolic and data-driven mathematical models for aerospace systems, circumventing traditional first-principles modeling limitations. Sparse Identification of Nonlinear Dynamics (SINDy) has shown promise in learning quadrotor dynamics from simulated data, offering a more efficient alternative. However, integrating methods like Dynamic Mode Decomposition (DMD) and Koopman operator theory further enhances model accuracy, particularly in capturing complex nonlinear dynamics while enabling optimal linear control. The approach is tested on UAV platforms with varying degrees of freedom, benchmarking it against neural network models such as ARX and NLARX for time-series data identification.
The research methodology employs key ML techniques, including transfer learning for adaptability across aircraft types, modular design for system flexibility, and domain randomization to enhance robustness. Experimental validation includes simulated environments replicating diverse flight conditions, with metrics such as accuracy, latency, and resource efficiency evaluated rigorously. SINDy and Koopman models undergo systematic refinement through data assimilation and statistical analysis to ensure reliability. Furthermore, integration efforts involve Hardware-in-the-Loop (HIL) simulation, adherence to industry standards like DO-178C, and risk assessment protocols to facilitate real-world applicability. By advancing ML-based modeling frameworks, this study aims to contribute to the next generation of aerospace control systems, optimizing efficiency while addressing scalability and measurement noise challenges

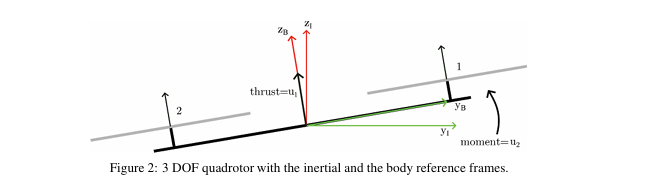